Images Recognition
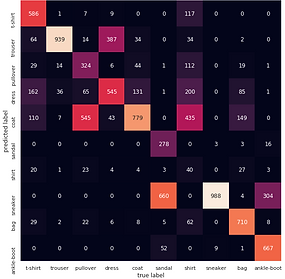
The MNIST dataset is a collection of images created by the National Institute of Standards and Technology (NIST). Each image in the dataset consists of 28 x 28 pixels. With the provided 60,000 images for training and 10,000 for testing, users have typically found between 93 to near 100% accuracy. The successful
implementation of varying models has been both a triumph and a problem for computer vision. Specifically, since the optimal solution has been attained, predicting handwritten images is no longer one of the defacto challenges.
To further expand on the earlier handwritten problem, the fashion MNIST dataset has emerged. Like the original MNIST dataset, the fashion variant consists of the same number of train and test images, each being the same dimension. However, each image in the collection is a series of ten possible clothing types.
In this study, the fashion MNIST dataset will be compared using neural network, Gaussian naïve Bayes, and Decision Tree Classifier. The analysis will primarily focus on comparing accuracy when predicting with either algorithm. In the future, additional algorithms can be tested, as well as overall benchmarking between each algorithm and prediction.
​
The dataset used for this study was obtained directly from the fashion-mnist.load_data straight from the cloud.
Since this study has been a simplified computer vision problem, no additional data scrubbing was performed. However, future studies could possibly improve the analysis by including additional images that are not the target fashion groups.
Utilized tenssorflow.keras to import the data sets, confusion matric for prediction verification, and classification report for classification accuracy.
​
Model
​
-GaussianNB
-Decision Tree Classifier
-Neural Network
​
Overall this study has succeeded in demonstrating how a multilayer neural network can outperform the GaussianNB and Decision Tree Classifier. However, as stated in the results, it would be interesting to explore the adjustment of parameters for both techniques. Specifically, adjusting the penalty and changing the kernel. It may or may not changes the result.
At a higher level, the fashion MNIST dataset is more interesting than its predecessor. More improvements and additional techniques will be needed to achieve the same or close level of accuracy as the numbers example.
​
​
​
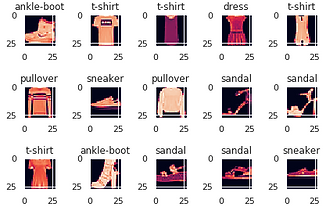
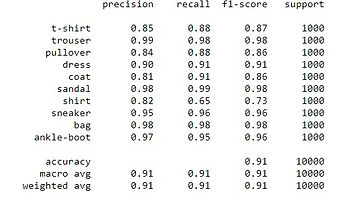